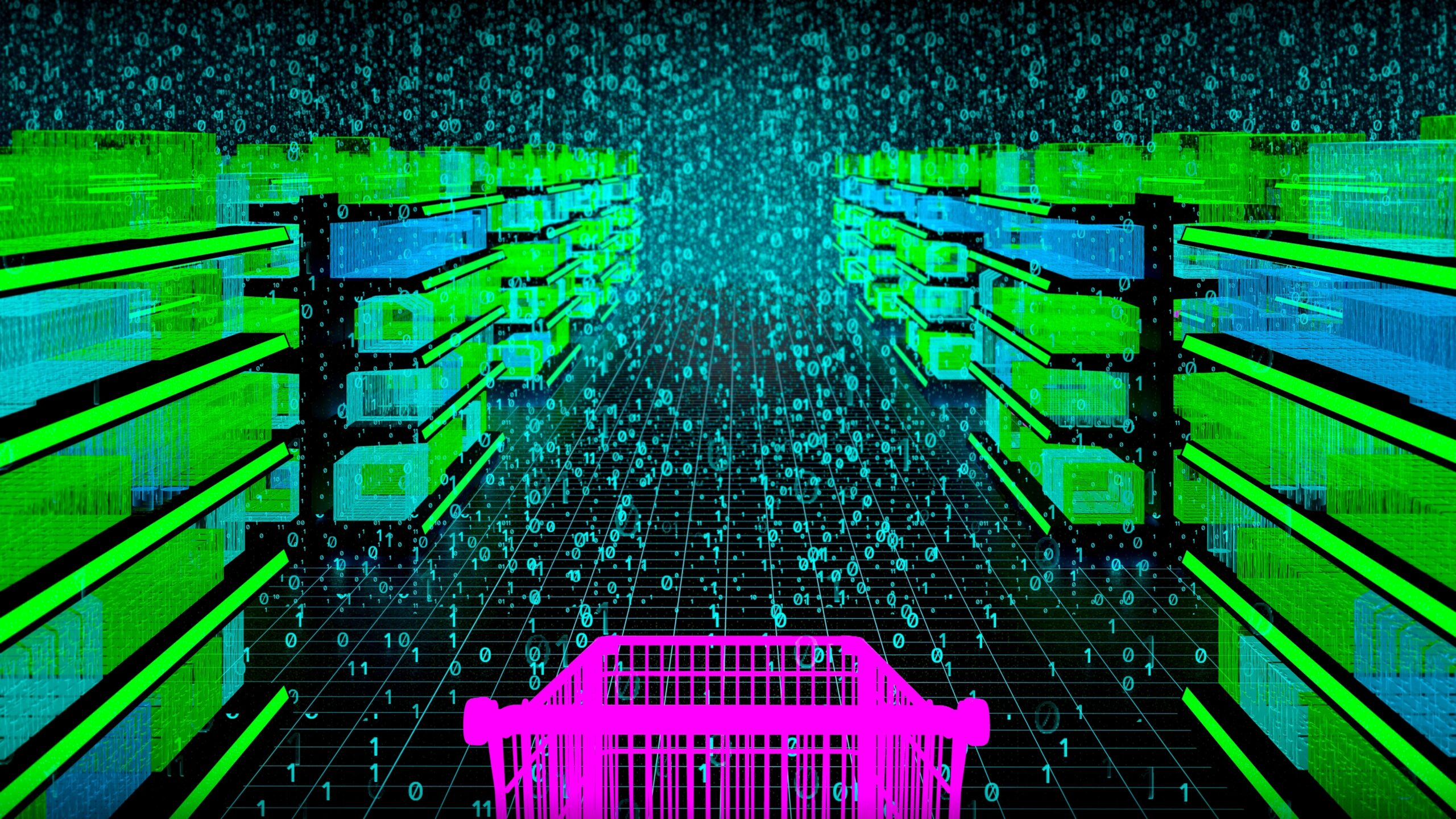
Objective
In an article published by Retail Touchpoints, 74% of brick-and-mortar shoppers said the checkout process was the biggest pain point in their retail experience. As such, this was only further confirmation for a leading U.S. retail corporation to explore the implementation of machine learning and frictionless checkout technology across their small footprint grocery stores.
Method
InContext was commissioned to provide high volume 3D modeling and related services for shelf stable, frozen, and select perishable inventory at one the retailer’s small footprint grocery stores.
To fulfill the scope of the project, InContext performed significantly scaled product acquisition, captured required item attributes, executed studio quality photography, and created and delivered over 29,000 3D models. These 3D model outputs were then used as machine learning inputs to enhance object recognition in effort to train store cameras on how to validate items being sold. This would ensure shoppers were charged appropriately for the items placed in their baskets when exiting the store.
Results
With an ecosystem designed for quality and scale, InContext was able to deliver on a scope of work that required flawless execution with no margin for error in 9 weeks. 3D product models were delivered as they were completed, which averaged out to a weekly throughput of nearly 3,500 models.